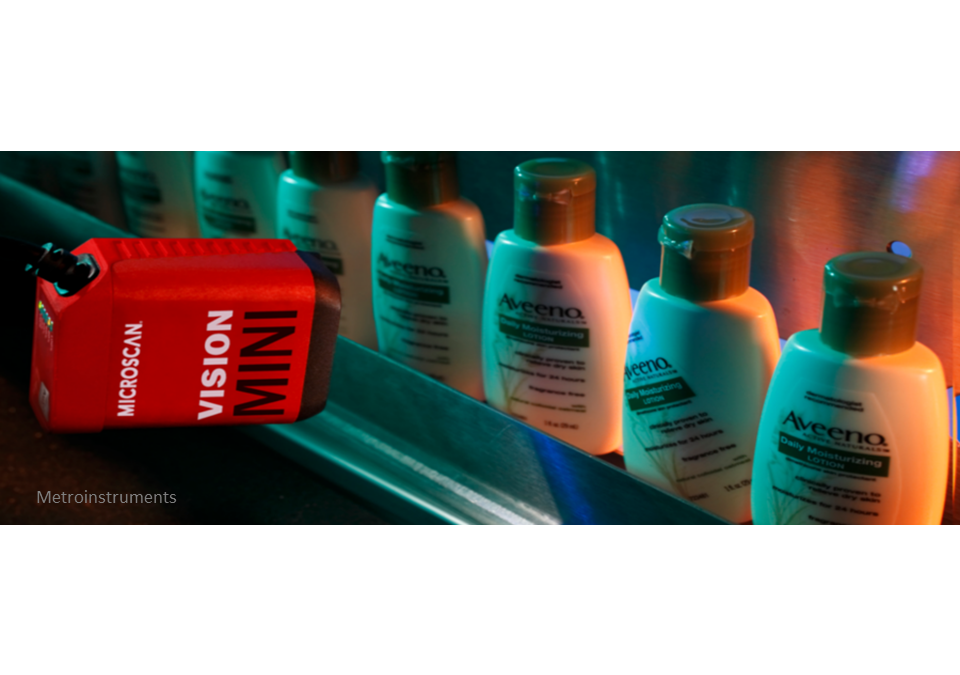
Machine vision for quality control
Machine vision is behind many of the great advances in the automation of the industry since it allows the control of quality of 100% of the production in processes with high cadences.
A non-automated process can be inspected by the operators themselves in the production process. However, in a highly automated process, inspecting the total production manually is a really costly process. Sampling inspection, i.e. determining the quality of a lot by analyzing a small portion of the production, has been used as a compromise solution, but due to the increasingly demanding quality demands of the final product, sampling inspection is not the solution.
It is in this context that the need to incorporate automatic systems for quality control arises, among which stands out the visual inspection through machine vision. The human ability to interpret images is very high, adapting easily to new situations. However, repetitive and monotonous tasks cause fatigue and therefore the performance and reliability of the operator’s inspection decline rapidly. One must also consider the inherent human subjectivity that makes two different people provide different results in the same situation. It is precisely these problems that can best address a machine, because it never tires, is fast and results are constant over time.
It is logical to think that the aim of a machine vision system is to emulate the virtues of people’s vision. For this, the first thing we must ask ourselves is, “what do we see with?” A simple question that common mortals would answer without hesitation “with the eyes”. However, the people who dedicate ourselves to machine vision would answer in a quite different way and say “with the brain”. Similarly, it can be thought that cameras are in charge of “seeing” in a machine vision system, when really that process is carried out by the image processing algorithms.
Obviously, in both cases it is a simplification of the problem, since the process of vision, natural or artificial, cannot be carried out without involving both eyes / cameras and brain / processing, without forgetting another key factor, illumination.
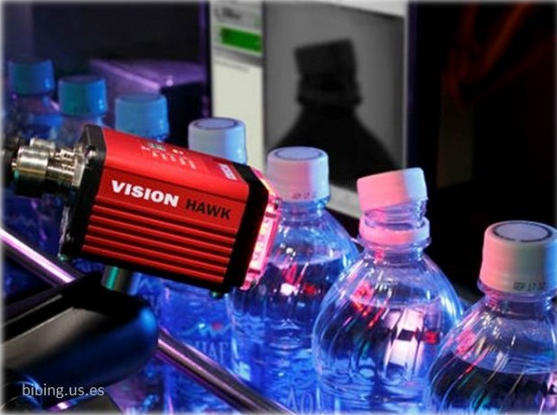
Many efforts have been made to try to emulate the human capacity to process images. This is why in the 1950s the term Artificial Intelligence (AI) was used to refer to the ability of a machine to display human intelligence. Among those capacities is that of interpreting images. Unfortunately, our knowledge about the functioning of the brain is still very limited, so the possibility of imitating such functioning is too. The development of this idea in the field of machine vision has been carried out by means of what is called Machine Learning (ML) popularized in recent years with the techniques of Deep Learning (DL) applied to the understanding of scenes. However, these techniques do not really have intelligence behind them, but rather are based on feeding them with a huge amount of images previously labeled by people. The processing that allows to classify the images as expected is considered like a black box and really, in most cases, we do not know why it works or not.
When machine vision is applied to the industry for the quality control there is usually not enough data to apply these techniques and it is required that the behavior of the system is always very predictable, so these techniques have not yet been popularized in the industry. That is why, when developing applications of machine vision for the industry, the objective is to solve well-defined problems in which cameras and lighting are selected to enhance the characteristics that are desired to be inspected in the image and subsequently endowed the system with the capacity of interpreting the acquired images with really low error levels.
Finally, the inspection results are stored and used in the production process, both to discard the units that do not meet the quality requirements before adding them a new value or to improve the manufacturing process and therefore reduce the production of defective units. This information is also used to ensure that the product met the quality conditions when it was delivered to the customer.
Among the different applications in which these techniques can be use are geometric inspection, surface finish inspection, the detection of imperfections in manufacturing, product classification, packaging control, color and texture analysis… and so on.
At CARTIF we have carried out numerous installations of machine vision systems such as cracking and pore detection in large steel stamped pieces for bodyworks, detecting the presence, type and correct placement of car seat parts, the detection and classification of surface defects in rolled steel, inspection of brake disks, detection of the position of elements for their depalletising, quality control of plastic parts or the inspection of the heat sealing of food packaging.