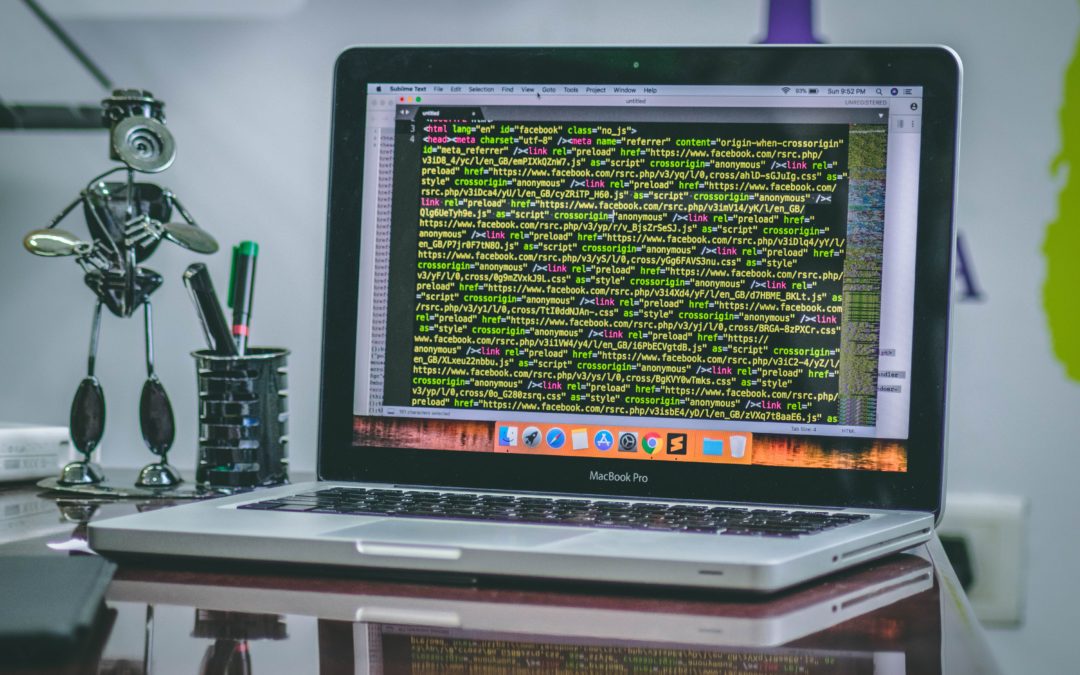
Artificial Intelligence and Intelligent Data Analysis: statistics and math, not magic!!
Artificial Intelligence, Machine Learning, Deep Learning, Smart Devices, terms that we are constantly bombarded with in the media, making us believe that these technologies are capable of doing anything and solving any problem we face. Nothing is further from reality!!
According to the European Commission, “Artificial intelligence (AI) systems are software (and possibly also hardware) systems designed by humans that, given a complex goal, act in the physical or digital dimension by perceiving their environment through data acquisition, interpreting the collected structured or unstructured data, reasoning on the knowledge, or processing the information, derived from this data and deciding the best action(s) to take to achieve the given goal.”1.
AI encompasses multiple approaches and techniques, among others machine learning, machine reasoning and robotics. Within them we will focus our reflection on machine learning from data, and more specifically on Intelligent Data Analysis aimed at extracting information and knowledge to make decisions. Those data (historical or streaming) that are stored by companies over time and that are often not put into value. Those data that reflect the reality of a specific activity and that will allow us to create statistical and mathematical models (in the form of rules and/or algorithms) that contain information about what reality is. Then, how to “cook” the data to obtain relevant information? What are the main actors involved? First the data, which will be our “ingredients”; second the algorithms capable of processing these data, which will be our “recipes”; third computer scientists and mathematicians, who will be the “chefs” capable of correctly mixing data and algorithms; and forth the domain experts, who will be our private “tasters” and whose task will be to validate the results obtained.
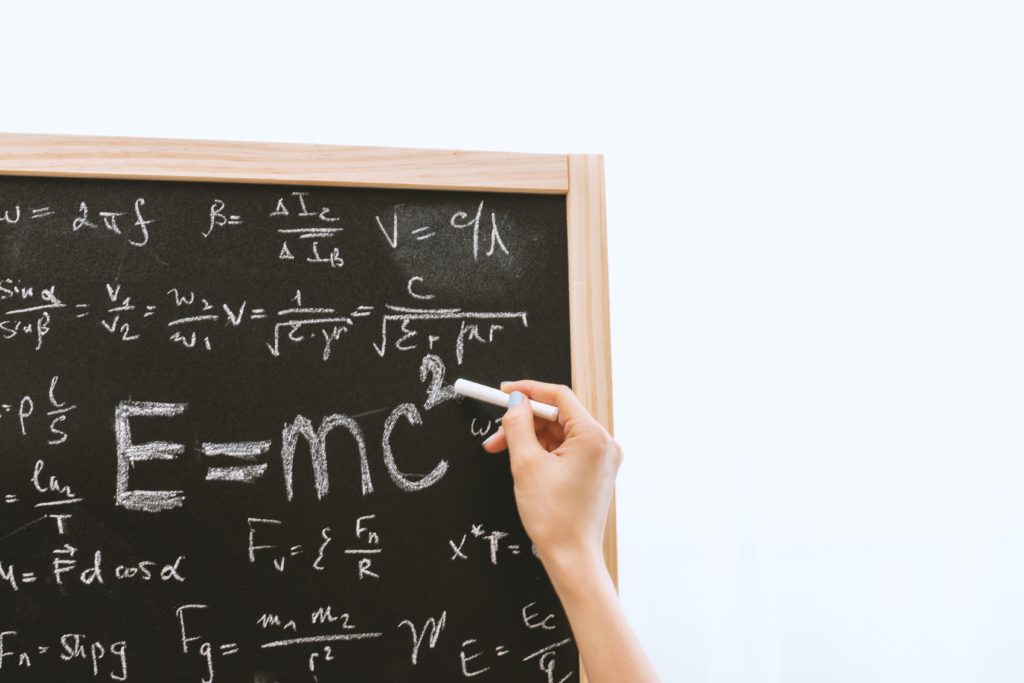
First one the data. Those data from which we want extract information in order to generate models or make predictions. Through a continuous learning process of trial and error, based on analysing how things were in the past, what trends there were, what patterns were repeated,etc. we can build models and make predictions that will be as “good” as data are. It is not a question of quantity, but of quality data. What does that mean exactly? It means that if we want to teach an AI system to multiply (giving it examples of correct multiplications) the system will know how to do that task (multiply) but it will never know how to subtract or divide. And if we give it ‘wrong’ examples (3*2=9 instead of 3*2=6) the system will learn to multiply, but in the wrong way. Therefore, as fundamental ingredient of our recipe, data must be well organized, be relevant and quality
On the other hand, the AI algorithms. Our “recipes” that tell us how to mix the “ingredients” correctly, how to use the available data to try to solve our problem. Algorithms that allow us to build computer systems that simulate human intelligence when automating tasks. However, not all algorithms can be used to solve any type of problem. On the “inside” of these algorithms there are mainly mathematical and statistical formulas proposed decades ago, and whose principles have advanced little in recent years, but which are now more effective thanks to (1) the increase in the amount of data and (2) the increase in power computer calculation (which is allowing much more complex calculations in less time and at low cost). However, skills such as intuition, creativity or consciousness are human abilities that (for now) we have not been able to transfer to a machine effectively. Therefore, our “chefs” and our “tasters” will be in charge of contributing these human factors in our particular”kitchen”.
That is why not all problems can be solved using AI. Because neither data are capable of “speaking” by themselves (they are not “carriers” of the absolute truth) nor are algorithms “seers” capable of guessing the unpredictable. What data and algorithms really know how to do is answer the questions we ask them based on the past, as long as the questions asked are the right ones. After the failure of a machine, how is the data provided by the sensors that monitor the machine mathematically related to the failure produced? When an image is analysed, how similar is it to images that have been previously analysed? When a question is asked of a virtual assistant, what answer has been given (by humans) more frequently in the past to that same question? It is therefore about questioning the data in the correct way so that they reveal the information we want.
Over the last century, AI has survived several technological ‘winters’ with lack of funding and research, mainly caused by the uncontrolled enthusiasm put into technology in the previous years2. It´ s time to “learn” from our hisorical data and not make the same mistakes again. Let´ s acknowledge AI for the capabilities it really has, and leave to wizards the ability to make the impossible come true. Only in this way AI will enter in its perpetual spring.
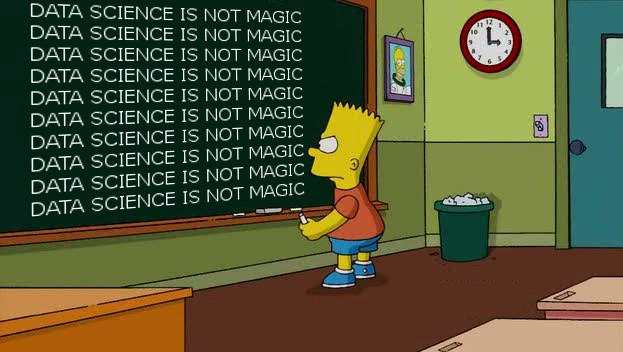
1 https://op.europa.eu/en/publication-detail/-/publication/d3988569-0434-11ea-8c1f-01aa75ed71a1
2 https://link.springer.com/chapter/10.1007%2F978-3-030-22493-6_2