The impact of Artificial Intelligence (AI) is highly recognized as a key driver of the industrial digital revolution together with data and robotics 1 2. To increase AI deployment that is practically and economically feasible in industrial sectors, we need AI applications with more simplified interfaces, without requiring highly skilled workforce but exhibiting longer useful life and requiring less specialized maintenance (e.g. data labelling, training, validation…)
Achieving an effective deployment of trustworthy AI technologies within process indsutries needs a coherent understanding of how these different technologies complement and interact with each other in the context of domain-specific requirements that industrial sectors require3, such as process industries who must leverage the potential of innovation driven by digital transformation, as a key enabler for reaching Green Deal objectives and expected twin green and digital transition needed for a full evolution towards circular economy.
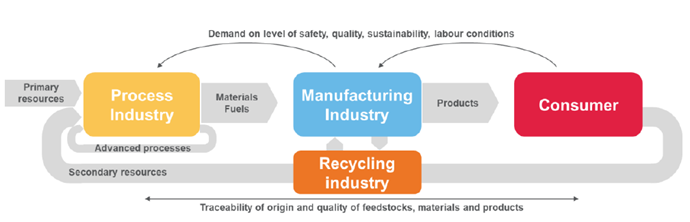
One of the most important challenges for developing innovative solutions in the process industry is the complexity, instability and unpredictability of their processes and impact into their value chains. These solutions usually require: running in harsh conditions, under changes in the values of process parameters, missing a consistent monitoring/measurement of some parameters important for analysing process behaviour and difficult to measure in real time. Sometimes, such parameters are only available through quality control laboratory analysis that are responsible to get the traceability of origin and quality of feedstocks, materials and products.
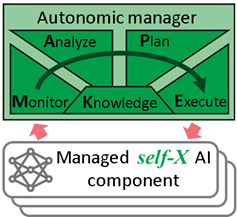
For AI-based applications, these are even more critical constraints, since AI requires (usually) a considerable amount of high-quality data to ensure the performance of the learning process (in terms of precision and efficiency). Moreover, getting high quality data usually requires an intensive involvement of human experts for curating (or even creating) the data in a time-consuming process. In addition, a supervised learning process requires labelling/classifying the training examples by domain experts, which makes an AI solution not cost-effective.
Minimizing (as much as possible) human involvement in the AI creation loop implies some fundamental changes in the organizations of the AI process/life-cycle, especially from the point of view of achieving a more autonomous AI, which leads to the concept of self-X AI4 . To achieve such autonomous behaviour for any kind of application it usually needs to exhibit advanced (self-X) abilities like the ones proposed for the autonomic computing (AC)5:
Self-X Autonomic Computing abilities
Self-Configuration (for easier integration of new systems for change adaptation) |
Self-Optimization (automatic resource control for optimal functioning) |
Self-Healing (detection, diagnose and repair for error correction) |
Self-Protection (identification and protection from attacks in a proactive manner) |
Autonomic Computing paradigm can support many AI tasks with an appropiate management, as already reported in the scientific community 6 7 . In AI acts as the intelligent processing system and the autonomic manager (continuously executes a loop of monitoring-analyzing-planning-executing based on the knowledge (MAPE-K) of the AI system under control for developing a self-improving AI application.
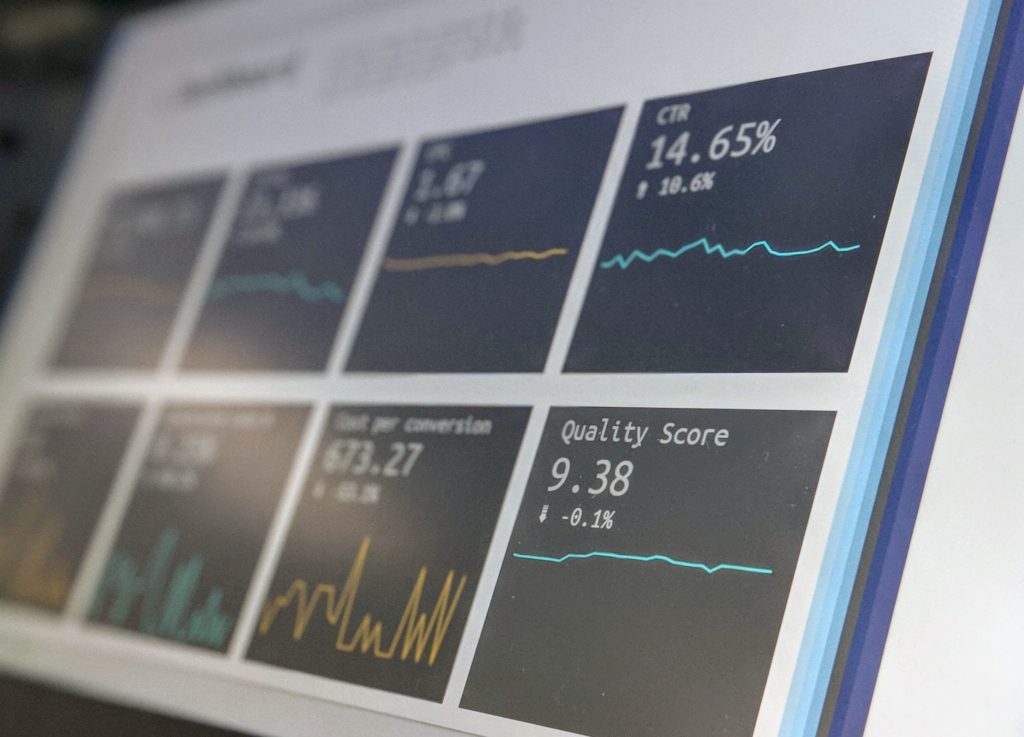
Indeed, such new (self-X) AI applications will be, to some extent, self-managed to improve their own performance incrementally5. This will be realized by an adaptation loop, which enables “learning by doing” using MAPE-K model and self-X abilities as proposed by autonomic computing. The improvement process should be based on continuous self-Optimization ability (e.g. hyper-parameter tuning in Machine Learning). Moreover, in the case of having some problems in the functioning of an AI component, the autonomic manager should activate self-Configuration (e.g. choice of AI method), self-Healing (e.g. detecting model drify) and self-Protection abilities (e.g. generating artificial data to improve trained models) as needed, based on knowledge from AI system.
In just a few weeks, CARTIF will start a project with the help of AI experts and leading companies of various process industry sectors across Europe to tackle these challenges and close the gap between the AI and automation by proposing a novel approach for a continuous update of AI applications with minimal human expert intervention, based on an AI data pipeline, which exposes autonomic computing (self-X) abilities, so called self-X AI. The main idea is to enable the continuous update of AI applications by integrating industrial data from physical world with reduced human intervention.
We’ll let you know in future posts about our progress with this new generation of self-improving AI applications for the industry.
1 Processes4Planet, SRIA 2050 advanced working version
2 EFFRA, The manufacturing partnership in Horizon Europe Strategic Research and Innovation Agenda.
3 https://www.spire2030.eu/news/new/artificial-intelligence-eu-process-industry-view-spire-cppp
4 Alahakoon, D., et al. Self-Building Artificial Intelligence and Machine Learning to Empower Big Data Analytics in Smart Cities. Inf Syst Front (2020). https://link.springer.com/article/10.1007/s10796-020-10056-x
5 Sundeep Teki, Aug 2021, https://neptune.ai/blog/improving-machine-learning-deep-learning-models
6 Curry, E; Grace, P (2008), “Flexible Self-Management Using the Model–View–Controller Pattern”, doi:10.1109/MS.2008.60
7 Stefan Poslad, Ubiquitous Computing: Smart Devices, Environments and Interactions, ISBN: 978-0-470-03560-3
- Behind the Curtain: Explainable Artificial Intelligence - 12 July 2024
- Digital Twin: Industry 4.0 in its digitised form - 9 October 2023
- Artificial Intelligence, an intelligence that needs non-artificial data - 16 December 2022