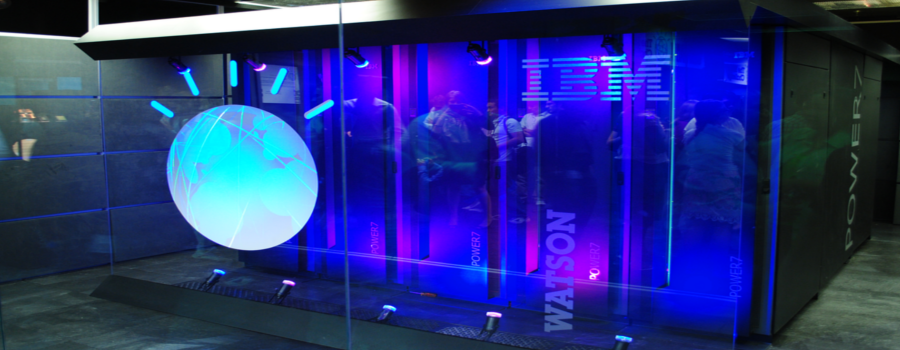
Is the Artificial Intelligence a threat for your job?
There is a growing concern caused by the possible effects Artificial Intelligence (AI) could have on everyday working life. Recently in the Davos Forum they have dealt with this issue, but two years ago The Economist published an article about the potential job lost that will be caused by this technology.
Films have made Artificial Intelligence familiar to everybody. From Colossus: The Forbid Project, where a super-computer managed to dominate the entire world and stole the girlfriend to its designer; to Ex Machina, where a heartless machine managed to fulfil its ambitions with no moral hesitation. Almost in all cases, it has been portrayed in a dystopian way.
However, the AI we will see soon will not look like an android, as Ava in Ex Machina, but it will resemble HAL 9000, the moral disoriented computer from 2001: A Space Odyssey. I think the first AI materialisation we will see is the Cognitive Computation, named by IBM as Watson.
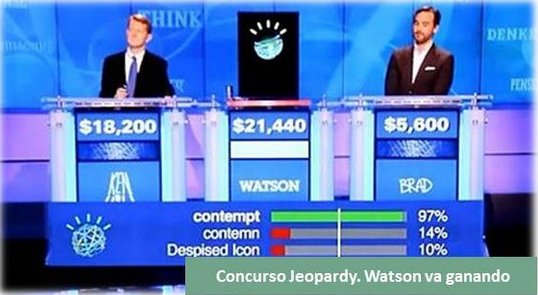
Watson is a machine able to answer questions posed in natural language capable of processing huge amounts of information to give the correct answer. It became known to general public in 2008, when it defeated two human opponents in Jeopardy!, a television contest featuring a quiz competition.
One of the first commercial Watson uses is to support lung cancer treatment by suggesting the best drug combination for every patient. Another application soon available will be to answer call phones in a call centre. Genesys, a company that develops and sells systems for that application, wants to include Watson in its portfolio. Watson will answer the phone, have a conversation with the user and refer him to a human operator if needed. The experience will be quite similar to the current one, but a machine will do a job that requires some intellectual abilities.
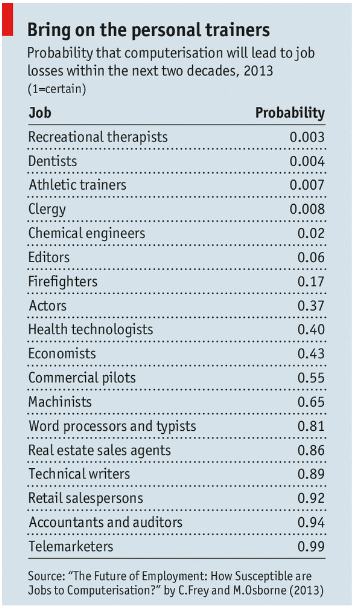
Aptitudes like the ones featured by Watson fear analysts there will be a job lost there where intellectual and routine tasks are done, even if qualification is needed as in accounting, layer assistants, technical writers or drivers. This is similar to fears arisen when the artificial force appeared: machines whose power enabled them to do the same work than a dozen people while they were driven by only one.
Technology has improved artificial force. While at the beginning it was powered by steam pressure, today it is enabled by automation and robotics. Artificial force ousted many workers and make some professions disappear but, at the same time, new jobs requiring higher qualification emerged. Workers had to do a transition from muscle to brain.
On the advent of this new Artificial Intelligence technology, able to carry out intellectual, repetitive tasks, how will be the new transition workers will have to do? It will have to aim at those tasks machines by the moment cannot do: creative and emotional jobs. However, the transition period could be not easy. Required formation could not be afforded by everyone, or to hire a machine could be cheaper than to hire a person. AI cost will be determinant and, considering only Watson hardware cost around three million dollars, it seems not every company will be able to access it.
In any case, we will have to face the old question: to let others to develop the technology and became mere users, or to be the scientific, technological or commercial developers of this new industrial revolution. A Hamlet like decision.