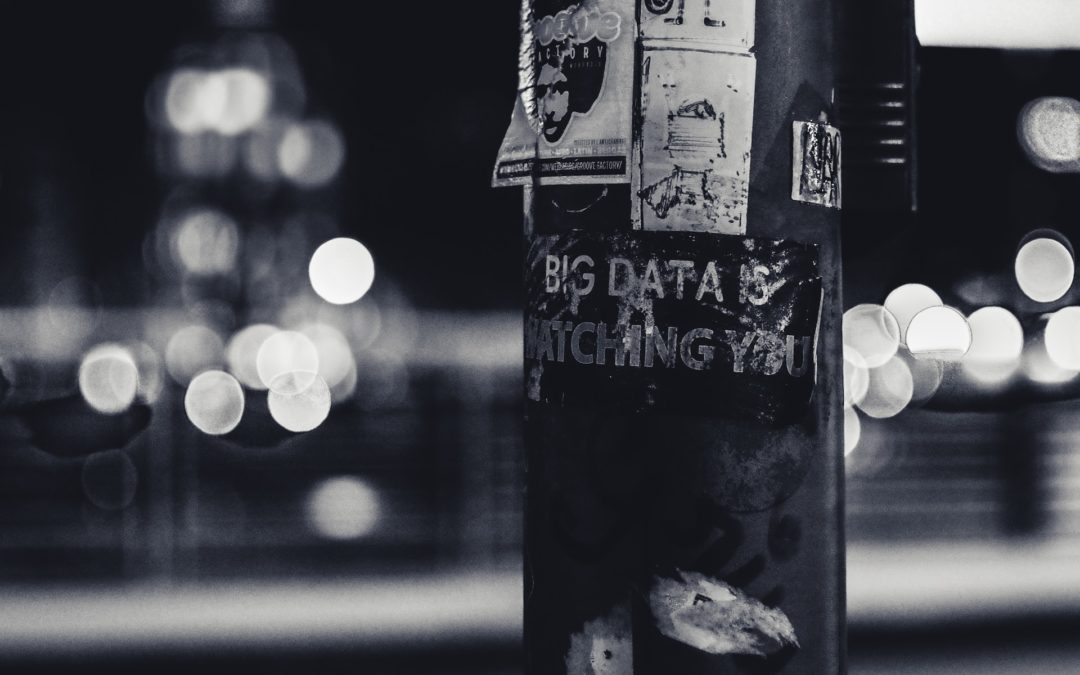
Managing industrial data: prevention is better than cure
In the field of health, it is known that is more effective prevent illnesses than treat them once they have manifested themselves. In a similar way, it can be apply in the context of industrial data, its continuous and proactive maintenance helps to avoid the need of an extensive pre-treatment before using advance data analytic techniques for decision-making and knowledge generation.
Pre-treatment data implies doing several tasks as: (1) data cleaning, (2) correction of errors, (3) elimination of atypical values and (4) the standardisation of formats, among others. These activities are necessary to assure quality and data consistency before using it in analysis, decision-making or specific applications.
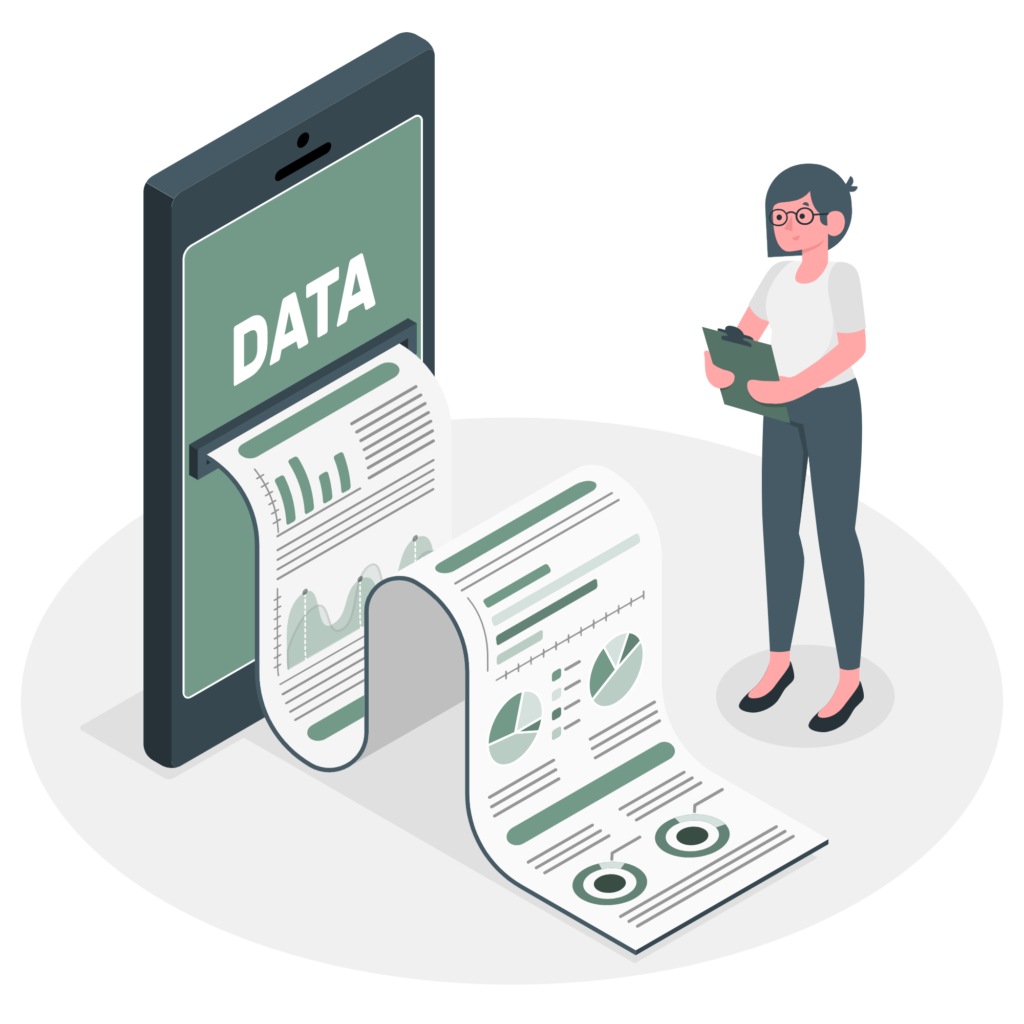
However, if robust data maintenance can be implemented from the outset, many of these errors and irregularities can be prevent. By establishing proper data entry processes, applying validations and quality checks, and keeping up-to-date records, it is possible to reduce the amount of pre-treatment need later, identifying and addressing potential problems before they become major obstacles. This includes early detection of errors such as inaccurate data, correction of inconsistencies and updating of outdated information. It is true that companies currently store large amounts of data but it is important to highlight that not all of this data is necessarily valid or useful, for example, for use in an artificial intelligence project. Indeed, many organisations face the challenge of mantaining and managing data that lacks relevance or quality. This management aims to ensure te integrity, quality and availability of data over time.
Efficient data maintenance is crucial to ensure that data are relaible, up-to-date and accurate, but this involves continuous monitoring and management by company staff, ensuring that they remain accurate, consistent, complete and up to date. The most common activities related to data maintenance include:
- Regular monitoring: Is carried out a periodic data tracking to detect possible problems, such as errors, inconsistencies, loses or atypical values. This can involves the revision of reports, tendance analysis or the implementation of authomatized alerts to detect anomalies.
- Updating and correction: If errors or inconsistencies in data are identified, maintenance staff will ensure that theyr are corrected and updated appropriately. This may involve reviewing records, checking external sources or communicating with those responsible for data collection.
- Backup and recovery: Procedures and systems are established to back up data and ensure its recovery in the event of failure or loss. This may include implementing regular backup policies and conducting periodic data recovery tests.
- Access management and security: Data maintenance staff ensure that data is protected and only accessible by authorised users. This may involve implementing security measures such as access control, data encryption or monitoring audit trails.
- Documentation and metadata update: Dara-related documentation, including field descriptions, database structure and associated metadat, is kept up to date. This facilitate the understanding and proper use of the data by users.
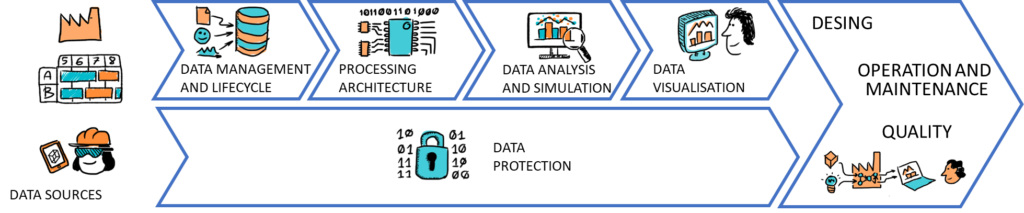
In summary, data maintenance involves: (1) regularly monitoring, (2) correcting errors, (3) backing up, and (4) securing the data to ensure that it is in good condition and reliable. These actions are fundamental to mantaining the quality and security of stored information.
At CARTIF, we face this type of problems in different projects related to the optimisation of manufacturing processes for different companies and industries. We are aware of the amount of time consumed in staff hours due to the problems explained, so we are working on providing certain automatic mechanisms that make life easier for those responsible for the aforementioned “data maintenance”. One example is s-X-AIPI project focused on the development of AI solutions with auto capabilities that require special attention to data quality starting with data ingestion.
CO-authors
Mireya de Diego. Researcher at de Industrial and Digital Systems Division
Aníbal Reñones. Head of Unit Industry 4.0 at the Industrial and Digital Systems Division