The common denominator of artificial intelligence is the need of available, good qualilty and real data to advance in the different procedures needed to create and train the models. Practical research in AI often lacks available and reliable datasets so the practitioners can try different AI algortihms to solve different problems.
In some industrial research fields like predictive maintenance is particularly challenging in this aspect as many researchers do not have access to full-size industrial equipment or there are not available datasets representing a rich information content in different evolutions of faults that can happen to an asset or machine. In addition to that, the available datasets are clearly unbalances as the norm for machines is that they operate properly and only few examples of faults appear during their lifetime.
It´s very important from the AI research point of view the availability of reliable and interesting data sources that can provide a variety of examples to test different signal processing algorithms and introduce students and researchers into practical application such as signal processing, classification or prediction.
The ideal situation for researchers and developers of artificial intelligence solutions is that everyone, to a certain extent, shares data. But sharing data cannot be seen only as a way to help other people, sharing research data can bring many advantages to the data donor:
- It´s part of good data practice and open science as it is encouraged to make data accesible together with the scientific papers generated.
- Cut down on academic fraud and prevent publications of studies based on fake data.
- Validate results. Anyone can make a mistake, if we share the data we used, other researchers could replicate our work and detect any potential error in our work.
- More sicentific breakthroughs. This is especially true in social and health science where data sharing would enable for example more studies in human brain as Alzheimer´s Disease and many others.
- A citation advantage. Studies that make data available in a public repository are more likely to receive more citations than similar studies for which the data is not made available.
- Best teaching tools based on real cases.
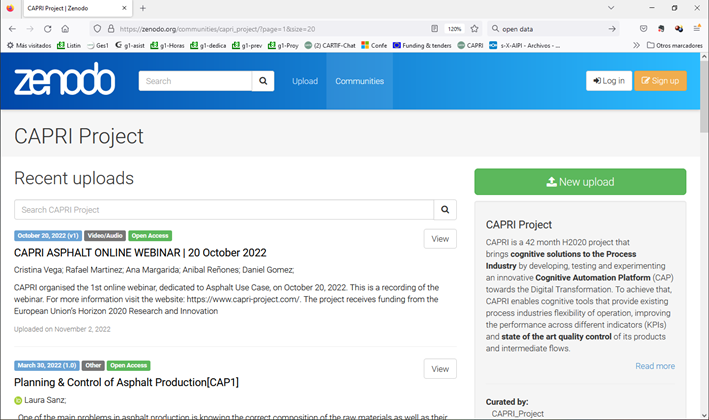
At Europe level the European Commission has launched the Open Research Europe, a scientific publishing service, for Horizon 2020 and Horizon Europe beneficiaries with a service to publish their results in full compliance with commission open access policies. The service provides an easy, high quality peer-reviewed venue to publish their results in open access, at no cost to them. Other interesting service part of this open research initiative is Zenodo, an open repository to upload your research results. In addition to the open research publishing guidelines, data guidelines are also available which adheres the F.A.I.R principles too and refers a number of trusted repositories like Zenodo, that we are obliged to use based on the European project rules.
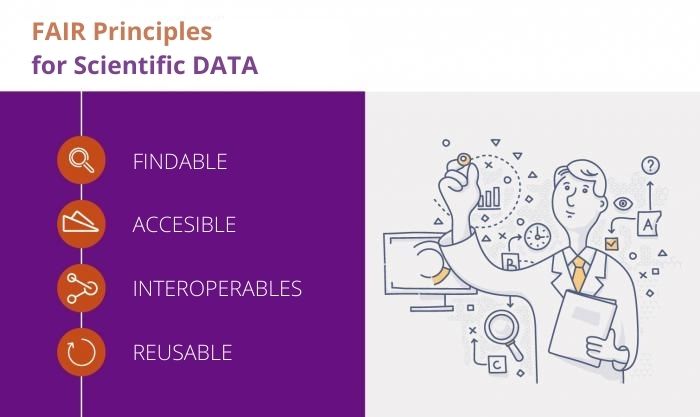
The FAIR guiding principles for publishing data mean that the data and its meta-data that defines it must be:
- Findable: (meta)data are assigned a globally unique and persistent identifier.
- Accessible: (meta)data are retrievable by their identifier using a standardized communications protocol.
- Interoperable: (meta)data use a formal, accessible, shared, and broadly applicable language for knowledge representation.
- Reusable: meta(data) are richly described with a plurality of accurate and relevant attributes.
Besides, from the governmental point of view European Commission, both European Data Strategy and Data Governance policy are powerful initiatives focus on the implementation of European data spaces, among which the Commission proposes the creation of a specific European industrial (manufacturing) data space to take advantages of the strong European industrial base and improve their competitiveness.
As researchers in CARTIF, we are committed to promote such openness with our research projects. For example, for CAPRI project it has been recently created its own Zenodo channel repository, where we periodically upload project results of the advanced solutions we are developing for the process industry such as cognitive sensors or cognitive control algorithms. You can go to the repository and inspect more than 40 datsasets, sourcecode or videos we already have uploaded.
- Behind the Curtain: Explainable Artificial Intelligence - 12 July 2024
- Digital Twin: Industry 4.0 in its digitised form - 9 October 2023
- Artificial Intelligence, an intelligence that needs non-artificial data - 16 December 2022